Blog post by:
Arcangelo Leone de Castris, Research Associate, The Alan Turing Institute
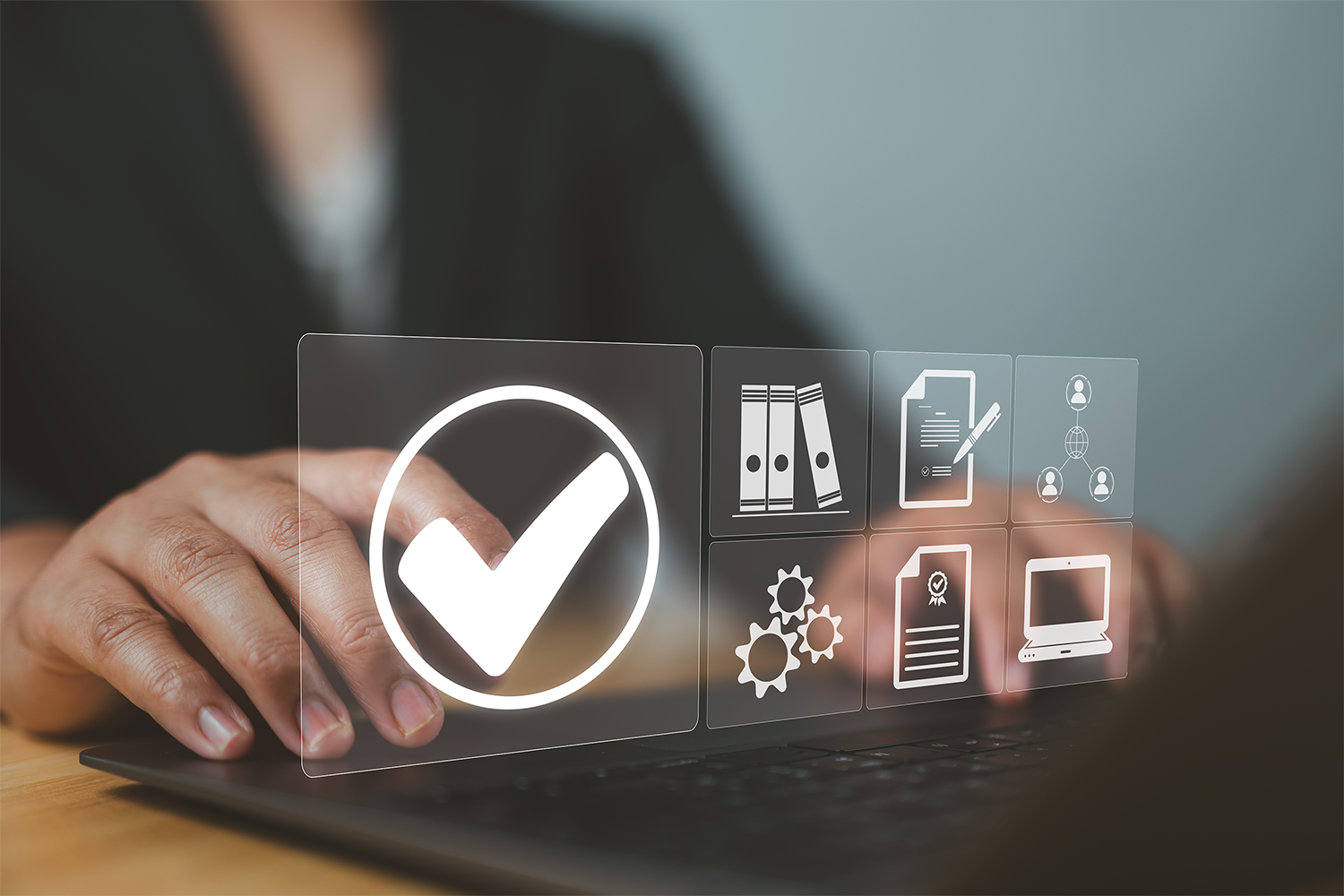
Organisations are investing in AI technologies to increase their productivity and deliver better products and services to their customers. From data analytics and decision-support systems to process control, supply chain management, and marketing ā companies can use AI technologies to support a wide range of tasks and processes. Ā
While AI systems can offer significant benefits, their adoption requires addressing complex challenges related to their safety, security, robustness, fairness, and accountability. If not adequately managed, these challenges can limit organisationsā ability to align AI systems with their goals and can lead to various types of harm stemming from inherent technical flaws, misuse, and abuse. Deploying AI systems in a safe and trustworthy manner requires establishing adequate governance frameworks, including risk management processes to ensure that relevant risks are identified, evaluated, and managed throughout the entire AI systemās lifecycle.Ā
Due to the relative novelty of AI technologies and the context-specific nature of the challenges they pose, it can be difficult to identify the full range of relevant risks. Additionally, as AI technologies and their capabilities evolve, new risks and challenges emerge. Organisations can take account of the versatile and dynamic nature of AI risks by setting up iterative risk identification processes covering the whole lifecycle of AI systems and taking into consideration the specificities of each AI use case. While some AI risks are unique to specific use cases, many AI applications share a set of baseline risks. As such, a systematic and shared understanding of the most significant and common risks organisations should consider when adopting AI technologies is crucial for helping them identify at least some of the risks relevant to their AI systems.Ā Ā
With the aim of contributing to more effective risk identification, auditing, and treatment, this blog proposes a harmonised, high-level taxonomy of AI risks for organisations. The proposed taxonomy maps the main sources of AI risk to specific harms and is based on a comparative analysis of four AI risk management frameworks: ISO/IEC 42001:2023 ā AI management systemāÆand ISO/IEC 23894:2023 āāÆGuidance on AI risk management; the NIST AI risk management framework; and the AI safety governance framework published by the Standard Administration of China (SAC). The taxonomy is also informed by the ongoing work of Cen-Cenelec JTC 21 to develop a risk management standard supporting the implementation of the EU AI Act and by other AI risk taxonomies found in the relevant literature such as the MITās AI Risk Repository and the AVID Taxonomy of AI risks.Ā Ā
Defining AI riskĀ
Based on the frameworks analysed, two different approaches to defining AI risk can be distinguished.Ā Ā
āOrganisationalā approaches to risk management focus on ensuring that products work as expected and frame the definition of risk from the perspective of the organisation. Frameworks that adopt an āorganisationalā approach often define risk as the effect of uncertainty on the objectives of the organisation. In this sense, provided such an effect can be positive or negative, risk can result in both threats and opportunities. Examples of frameworks that adopt this perspective are ISO/IEC 42001:2023 āāÆAI management system and ISO/IEC 23894:2023 āāÆGuidance on AI risk management, both consistent with the definitions provided by ISO 31000:2018, Risk management ā Guidelines and ISO/IEC 22989:2022 ā Artificial intelligence concepts and terminology. An alternative definition which also adopts the organisational perspective is provided by the NIST AI RMF. This framework defines risk as the composite measure of an eventās probability of occurring and the magnitude of the consequences of the corresponding event.Ā Ā
āProduct safetyā approaches, on the other hand, frame risk as the potential harm that products can cause to individuals and society. For example, both ISO/IEC Guide 51:2014 āāÆGuidelines for the inclusion of safety aspects in standards and the EU AI Act define āriskā as the ācombination of the probability of an occurrence of harm and the severity of that harmā where āharmā refers to damages to health, property, and environment, or interference with the fundamental rights of the individual. This definition will likely be adopted also by the European standards supporting the implementation of the Act.Ā
While the two approaches are closely related, methods required to ensure that a product functions as intended may differ from those needed to prevent it from causing harm to individuals or society. As such, different approaches to defining risk may lead to different risk management strategies. This blog considers both perspectives and tries to harmonise them to lay out a general taxonomy of AI risks for organisations.Ā Ā
AI systems and their application: Common sources of risk and related hazardsĀ
As innovation advances and AI technologies become more sophisticated and complex to manage, companies that develop and deploy AI systems face heightened exposure to an increasingly broad range of risks. AI risks can emerge at every stage of the AI value chain and depend on different combinations of technical and societal attributes of the technology. For instance, risk-determining factors include how a system is designed, trained, and tested, how it is used, whether it interacts with other AI systems, who operates it and for what purpose, how autonomous it is, and in what context it is deployed.Ā Ā
To better understand the complex risk profile of AI systems, it is useful to think in a structured and systematic way about AI risk sources and related hazards. Risk sources exist at both the technical and application levels of AI systems. At the technical level, risk sources can relate to the data used by the system, its algorithmic architecture, and other software and hardware components on which the system operation relies. At the application level, risk sources relate to the interaction between the system and its operational environment.Ā Ā
Data-related sources of risk, for instance, include the possibility that data are collected and used in illegitimate ways. This can lead, among others, to privacy and IP violations. Additionally, poor-quality datasets can raise the risk of inaccurate, biased, and discriminatory outputs, and datasets containing illegal or harmful content can cause the AI system to produce equally illegal or harmful results. Another risk source is data security. For example, data can be compromised by malicious actors at the point of collection ā a phenomenon known as data poisoning ā with the effect of degrading the performance of the AI system processing those data.Ā
Risk sources related to the algorithmic architecture of the system ā also referred to as āAI modelā ā include low degrees of transparency and explainability of the model that can lead to control and accountability issues, errors and bias embedded in the algorithmic design which can lead to inaccurate and discriminatory outcomes, and to security vulnerabilities. Core algorithm information such as weights, structures, and functions can be targeted by various types of adversarial attacks aimed at stealing information or affecting the model performance. Examples of these attacks include model theft and inversion.Ā Ā
Other components of the AI system like the hardware and software infrastructure supporting the AI model operation can also pose risks. Vulnerabilities in the hardware and software infrastructure can enable malicious actors unauthorised access to the system leading to data breaches and service disruption. In addition, both hardware and software components can fail to perform as expected. Defective components, network failures, and memory loss are some examples of risk sources that can lead to downtime and the disruption of operations.Ā
Beyond risks strictly related to the different components of AI systems, many risks are also due to the interaction between these systems and their operational environment. Some of the most common sources of risk related to the deployment of AI systems include the complexity of the environment and its dynamic nature. The complexity of the environment defines the range of potential situations an AI system can face while in operation. Designing and developing an AI system in a way that factors in all the scenarios that the system may have to deal with is as important as it is challenging. When faced with a scenario they were not designed for, AI systems can behave in unintended ways, becoming less reliable and robust. In this sense, the more complex the operational environment, the higher the potential for harm. Furthermore, dynamic environments pose additional challenges insofar as they can evolve to a point where the data on which the model was trained cease to adequately represent the characteristics of the operational environment ā a phenomenon referred to as concept or model drift āāÆleading the AI system to become more prone to error.Ā Ā
Another risk factor is the level of automation of the system. Depending on the use case, the degree of autonomy with which a system is enabled to operate in the world can raise ethical and safety risks. One common measure to mitigate such risks is introducing some degree of external supervision. External supervision can be automated or manual. If the supervision is performed by another AI system, many of the risk sources seen above will be true for that system too. In cases where supervision is done manually by a human, different types of hazards can emerge. For example, elements related to the ability of human supervisors to understand and evaluate the outputs of the AI system, such as their skills and attention span, can be sources of risk. For cases where the operation of the AI system requires processing sensitive data, another source of risk relates to the possibility that data processing is done in illegitimate and harmful ways. Relevant risks include, once again, cases of data protection and IP violations.Ā Ā
The social context in which a system operates and the types of subjects it affects can also pose significant risks. AI systems deployed in sensitive or strategically important environments such as health, law enforcement, or defence, can pose more serious societal risks, including economic, environmental, physical, and psychological risks, as well as the risk of violating fundamental rights. These risks are not exclusive to AI systems deployed in sensitive or strategic contexts and are highly dependent on the specificities of each use case. Nevertheless, it is important to stress that the social context in which an AI system is deployed has an impact on the types and severity of harm that the system can cause. Similar considerations are true also for the types of individuals affected by the system. If the system can impact vulnerable individuals or communities, it will have a greater potential to cause harm, including to the health and safety of those individuals, their fundamental rights and mental well-being, and their socioeconomic status. Finally, risks can derive from the specific capabilities of an AI system. For example, an AI system capable of operating the actuator of a mechanical arm in an open environment will pose a significant risk to the safety of the individuals who may come into contact with the machinery. Furthermore, AI systems with highly advanced capabilities that can have a significant negative impact on society, such as by spreading disinformation at scale or causing public security threats, are considered to pose a āsystemic riskā.Ā
Mapping common AI risk sources and related hazardsĀ
There are at least two different angles from which organisations can think about AI risks. From a narrow and strictly internal perspective, AI risks can translate into three high-level types of harm: financial, reputational, and legal costs. Financial costs include direct financial losses and the disruption of business operations. Reputational costs can translate into the loss of consumer trust, negative public perception, and a strategic disadvantage vis-Ć -vis the competition. Finally, legal costs refer, among others, to the cost of litigation, fines, penalties related to non-compliance, and of being subject to investigations by regulatory bodies. The same risks that can result in financial, reputational, and legal costs for organisations can also negatively impact the social context in which organisations operate. They can cause harm to the health, safety, and fundamental rights of individuals, as well as to property, critical infrastructure, and the natural environment. For example, an AI system used to illegitimately process personal data will cause legal and reputational costs for the company and harm the fundamental right to privacy of the data subjects. As such, for their AI system to be truly safe and trustworthy, organisations should dedicate sufficient resources to identify and assess both types of risks. A holistic approach to identifying AI risk is also necessary to capture risks that would otherwise be more difficult to detect. For instance, a company focusing only on internal risks will be better placed to realise the threat of reputational or legal harms raised by an AI system that, despite being technically sound and economically profitable, can cause psychological harm (e.g., addiction) to some of the individuals it interacts with.Ā Ā
In an attempt to highlight the relation between the risk sources and the risks discussed in the previous paragraph, the table below offers a tentative and non-exhaustive mapping of AI risk sources to AI hazards. The proposed mapping aims to combine organisational and societal perspectives on the types of harm potentially caused by AI systems.Ā Ā
Ā | AI value chain levels | AI risk sources | AI hazards |
---|---|---|---|
Technical layer
|
|
|
|
Ā |
|
|
|
Ā |
|
|
|
Application layer
|
|
|
|
ConclusionĀ
Identifying relevant AI risks is key to successful risk management. However, considering the fast pace of technological innovation and the highly contextual nature of some AI hazards, risk identification can be challenging. To support this process and contribute to more responsible AI adoption, this blog proposes a general taxonomy of AI risks from the perspective of organisations. More specifically, this blog maps different types of AI risk sources to specific hazards, intending to provide a structured and systematic overview of key elements to consider when adopting AI systems in an organisation. The proposed taxonomy is not exhaustive and represents the first step of a larger project aimed at mapping AI use cases to relevant risks and risk mitigation strategies.Ā
0 Comments