Blog post by:Ā
Sam Bilson, Senior Scientist, NPL
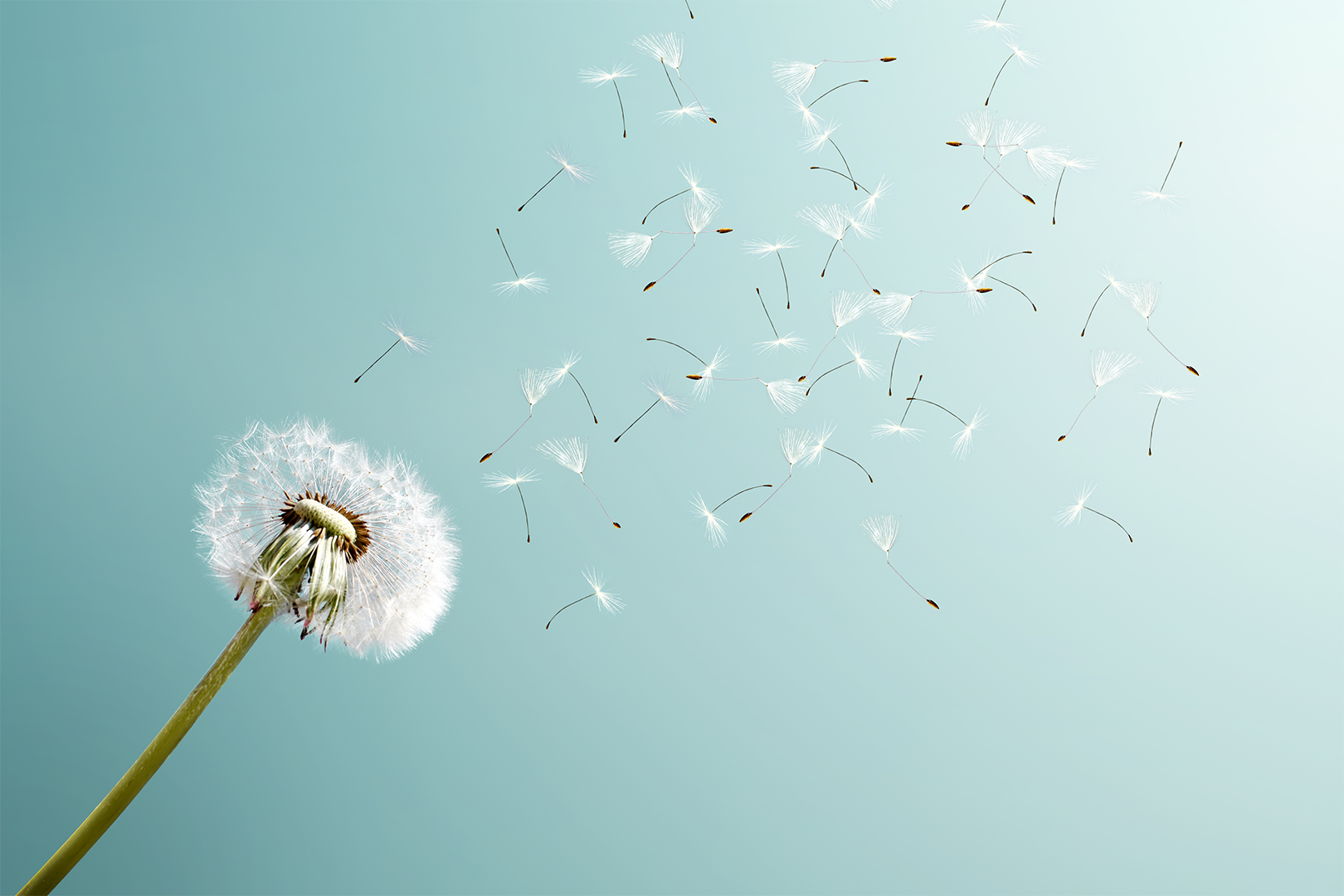
Hay fever blights the lives of many people in the summer. Fortunately, there are networks of monitoring stations across the world that detect pollen and fungal spores, enabling researchers to develop predictive models of the number of grains and spores released at different times of the year. These models, combined with the weather predictions of bodies such as the Met Office, can provide an estimate of the likely āpollen countā and help sufferers take remedial action.
The devices that detect pollen and count the number of particles use a variety of different approaches to get their estimates. The more traditional devices pull air into a machine containing a sticky surface which traps any airborne pollen or spores. The surface rotates such that only a portion of it is accessible at a given time, so that it is possible to deduce which part of the surface was exposed when and hence know which samples of pollen were trapped at different times. The sticky surface is removed from the machine and the pollen collected is taken to a laboratory to be viewed under a microscope. The grains are then counted and assigned to a taxon which identifies which plant (or fungus) the pollen (or spore) came from.
Historically, this task has been undertaken by a human expert. This process is time-consuming, and often involves analysis of a small sub-sample of the available data due to time constraints (this may lead to a large uncertainty associated with the measured value). Other types of measurement are being developed that can speed up the data capture and reduce uncertainty, but these approaches will require automated data analysis for efficient deployment.
This kind of identification and classification task is exactly the kind of job that machine learning (ML) is well-placed to automate. It is therefore unsurprising that pollen detection instrument manufacturers are developing algorithms that can identify and classify pollen grains. These approaches are set to revolutionise the field of bioaerosol monitoring. However, if they are to be used in practice then the measurements, including the data analysis and classification, must be well-understood and standardised.
ML also offers the chance to develop algorithms that addresses the challenges of geographical variations in pollen taxa and ambient conditions (pollen can be strongly affected by humidity). It does this by using transfer learning to develop locally tuned versions of a master algorithm rapidly but again the effects of this approach must be well understood before being deployed in practice.
A European project, funded by the European Metrology Programme, that addresses this need for standardisation has recently begun. The BioAirMet project brings together metrology institutes, research organisations, and instrument manufacturers to develop techniques for validation of AI algorithms for pollen classification. This includes methods for uncertainty propagation and for sensitivity analysis to understand how the characteristics of the training data affect algorithm performance. The project team will then feed their findings back to two relevant standards committees. The work will contribute to the development of a new standard on automatic pollen and fungal spore monitoring within the CEN/TC 264/WG 39 ā Sampling and Analysis of Airborne Pollen Grains and Fungal Spores, and will contribute to the revision of existing standards published by CEN/TC 264/WG 28 ā Measurement of Airborne Microorganisms in Ambient Air.
0 Comments