Blog post by
Professor Mark Levene
Principal Research Scientist, NPL
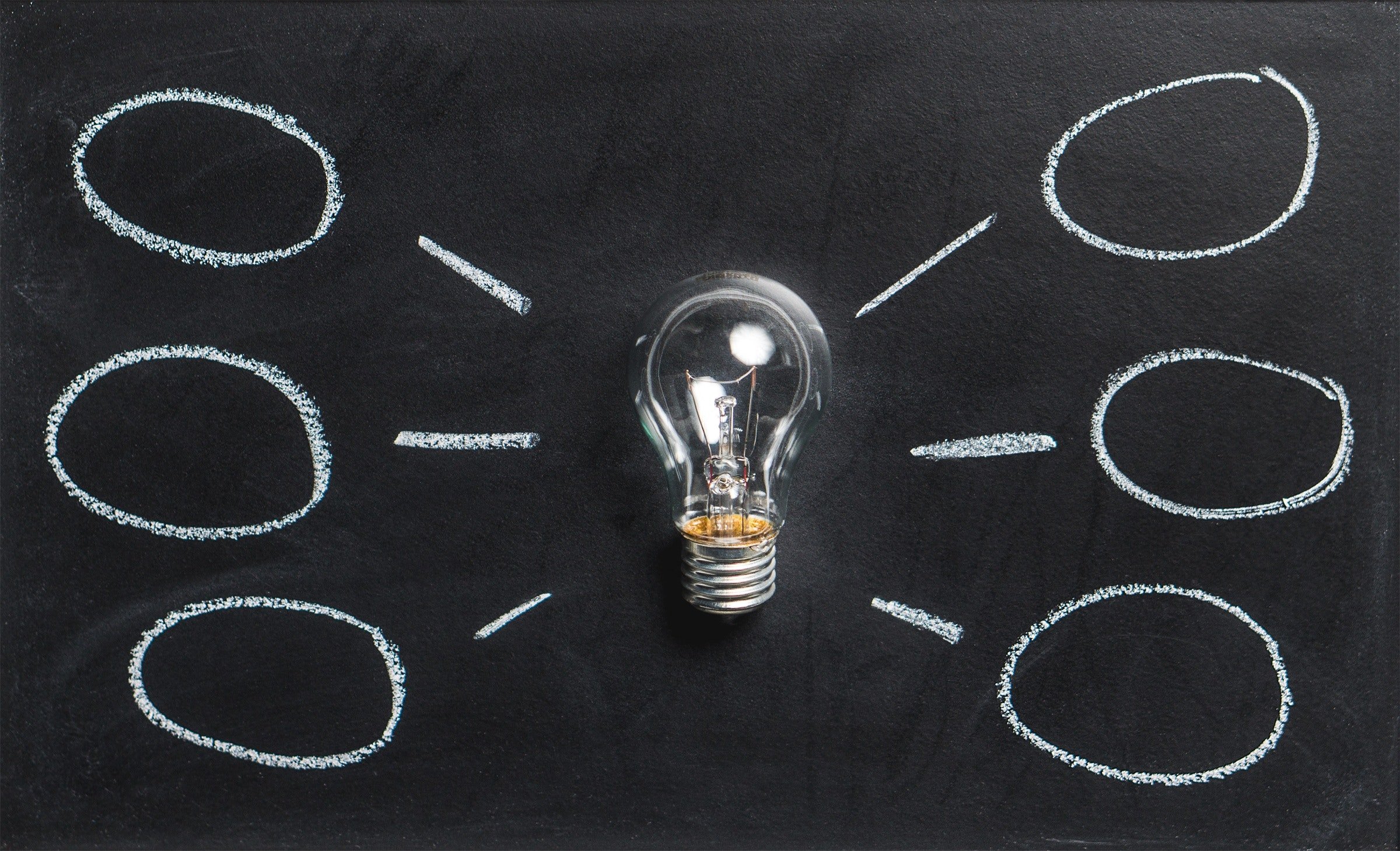
The purpose of ISO/IEC 42001 is to guide organisations on how to manage their AI (artificial intelligence) systems. This is important since the use of AI and ML (machine learning, which is a subset of AI of fundamental importance) raises several questions, including those regarding:
- The relative transparency and explainability of automated decision systems.
- The use of outputs such as data analysis from ML systems, which are trained from data, once or continuously, and adapt to changes in its inputs. This differs from traditional procedural programming, since an AI system may change its behaviour over the course of its use.
- The degree of autonomy of an AI system, as in autonomous driving vehicles.
In the Data Science Department at the National Physical Laboratory (NPL), we are investigating AI and ML systems in the context of metrology (the science of measurement), which can provide accurate measurements underpinning our confidence in the data used by such systems.
Defining Trustworthiness
In a broad sense an organisation should aim for their AI systems to be trustworthy. One may consider a person to be ātrustworthyā if that person is reliable, responsible, and dependable. For AI systems the notion of trustworthiness is more complex and includes ethical, technical and risk related components.
There is a technical report, ISO/IEC TR 24028, which deals with some of these issues. However, to have a better understanding of trustworthy AI it is important to look at publications from several sources, as there is no definitive agreement on its precise definition.
Managing AI systems using ISO/IEC 42001
The emphasis of ISO/IEC 42001 is on integrating an AI management system with the organisationās existing structures. In the standard, a management system is defined as āinterrelated or interacting elements in an organisation to establish policies and objectives, as well as processes to achieve the objectivesā.
To capture the components of a management system, the body of the standard is relatively generic, and we are referred to the standardās annexes as well as other ISO/IEC documents to get the AI perspective. One way to look at it is a tick list for an organisation relating to their AI system. Much of this is exactly what an organisation should be doing for the management of all their computer systems, regardless of whether it contains an AI component. An interesting point is that ML is not mentioned in the body of the standard, rather this is left to the annexes.
ISO/IEC 42001 for governance and trust
The standard has four annexes. Trustworthy AI is mentioned in annex A as part of the management guide for AI system development. In annex B, which deals with the implementation guidance for AI controls, there is further mention of specific measures pertaining to AI/ML. (A control is a measure that maintains and/or modifies risk.) In particular, it is mandated that documentation of data used in the organisation should include the categories used for ML, and the process of labelling the data for training and testing.
In terms of assessing the impact of AI systems on groups and individuals, the standard mentions several areas of trustworthiness such as fairness, transparency, explainability, accessibility and safety. Many other important areas of impact are mentioned such as the effect on the environment, potential misinformation, and possible adverse safety and health issues. However, these are relevant to software systems in general and not just to AI systems.
An interesting control is to provide justification for the development of an AI system, including an explanation of when and why the system will be used and a list of metrics that should be used to measure whether the systemās performance is compatible with these objectives. A question arising from this is whether known metrics that are used for software systems are sufficient for systems that contain AI.
In addition, the design choices need to be documented, which includes detail on the ML method. In this respect evaluation of the AI system is also important and will include AI specific measures.
Although not mentioned in the standard, generalisability is very important for AI systems, that is how does the statistical model built by the ML methods employed adapt to new data that was previously unseen. For deployment the model built by the machine learning may need to be periodically, or even continuously, retrained. Therefore, the AI system needs to monitored on an ongoing basis to assess the effect the systemās performance.
Data management and responsible AI
Annex B also addresses the data management processes that should be put in place. These cover specific guidance for AI systems that include transparency, explainability, and sample training data. It also includes guidance for data preparation, which includes statistical exploration of the data, a fundamental activity in data science. Responsible use of AI is also addressed, the objective being that the AI system should be trustworthy along multiple dimensions including fairness, accountability, transparency, reliability, robustness, safety, privacy, security, and accessibility.Ā
The dimensions of trustworthiness also apply to the subject of annex C, which addresses AI-related organisational objectives and risk sources.
Finally, annex D is about the domains and sectors in which an AI system may be used. The problem addressed is the integration of sector specific standards with this general AI standard. Annex D also deals with certification, pointing to an approach for third-party conformity assessment on the basis of the AI management standard.
As a whole ISO/IEC 42001 offers organisations both the guidance for their existing technical stacks as well as the standards and processes needed for the AI deployment built atop them to be trustworthy, along its various dimensions, some of which were mentioned above.
Trustworthy AI is one of the main themes we are researching at NPL, as it is of prime importance to ensure that any measurement system that includes an AI component adheres to the underlying principles of responsible AI. In a future blog post, we will look more closely at how ISO/IEC 42001 might be useful for an organisation looking to benefit from using AI and machine learning in their operations.
0 Comments