Blog post by:
Sundeep Bhandari, Head of Digital Innovation at NPL
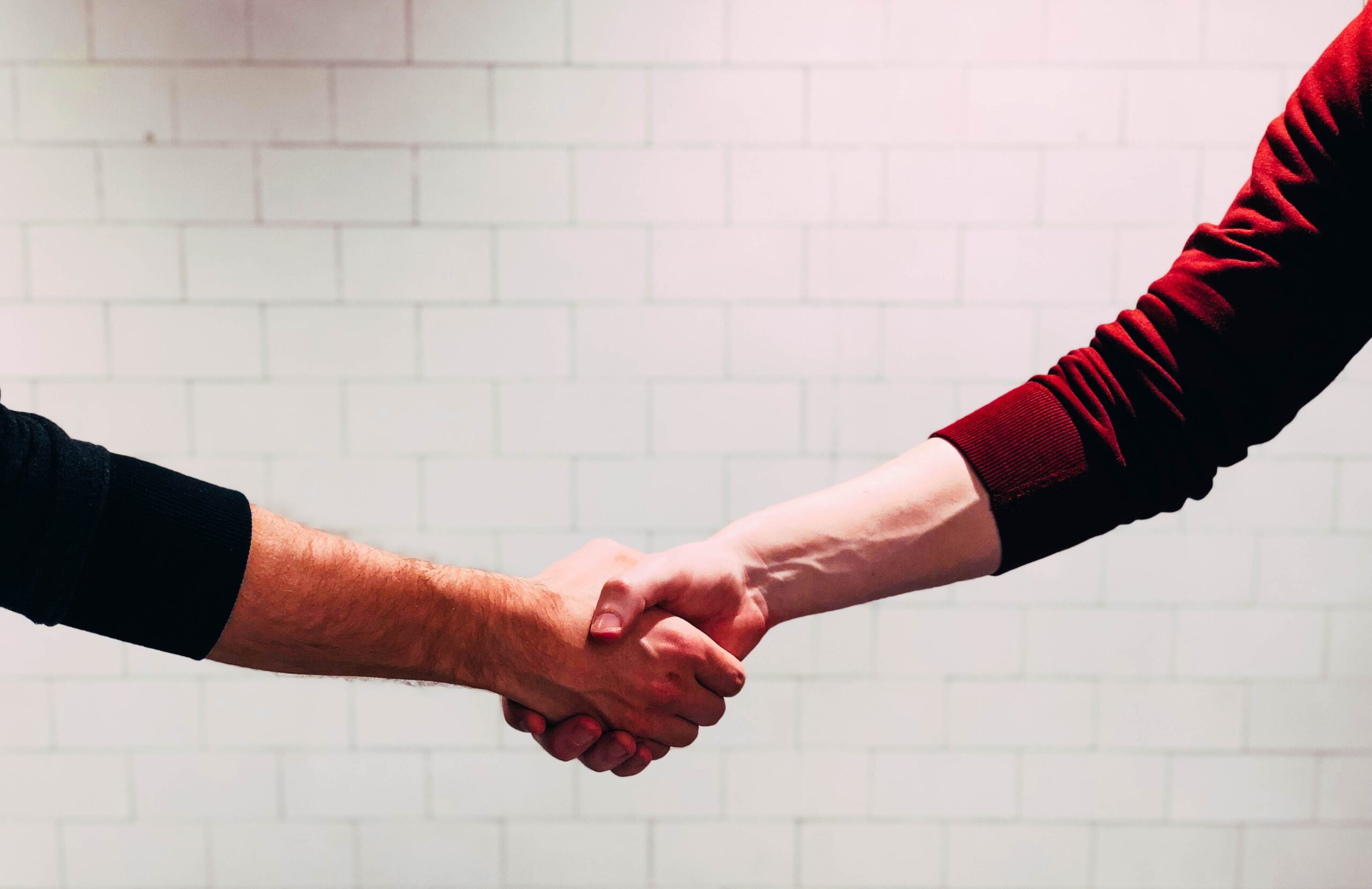
The global measurement infrastructure is the foundation upon which everything from academic research to entrepreneurial activity depends. It provides consumers and businesses with the confidence to engage in manufacturing, innovation, investment, trade, and even travel. There is one National Metrology Institute (NMI) in each country, responsible for maintaining that countryās national measurement standards and providing traceability to the International System of Units (the SI) at stated levels of confidence ā often called measurement uncertainty. The National Physical Laboratory (NPL) is the UKās National Metrology Institute. Measurement can be considered an invisible utility, and for over 100 years, our community has supportedĀ societies and economies.Ā
The role of an NMI is also to advance measurement science to ensure that the measurement infrastructure of the world remains relevant to societal needs. As the world transforms around us, we need to transform with it, and indeed stay ahead of it. Whilst physical infrastructure technologies have been in place for over 100 years, in this world increasingly driven by data at the speed of AI, the same cannot be said of the assets required to establish a digital measurement infrastructure.
Almost all of the āgame changingā products, solutions, applications and services ā across sectors and society ā that will help us address major global challenges (such as climate change, public health, energy resilience, and so on) will rely on confidence in data and its employment in advanced, AI-driven digital technologies. However, as AI systems require less and less human intervention, great care must be taken to assess or quantify the quality of AI systems and their subsequent impact on the users of these systems.Ā
Traditionally, NMIs have focused on quantification and quantitative inputs and outputs and providing uncertainty budgets (confidence levels), which are essential for output measurements to be considered trustworthy. However, evaluating and measuring in a world of data and AI requires us to consider additional approaches to supplement traditional metrology paradigms, to create verify and standardise the measurements and metrics that enable the evaluation of AI.
Therefore there is growing recognition that the approaches and the advancement of measurement science for trustworthy AI should be discussed and developed with both quantitative and qualitative characteristics in mind.Ā Ā Ā
Measurement of trustworthy AI is likely to be based upon the level of confidence we have in several characteristics that build trust. However, for AI these will be very context specific, hence approaches at the NMI level will require flexible use of qualitative and quantitative characteristics when evaluating AI systems. This demands an alternative approach, accounting for human factors and socio-technical aspects that address the interactions between people and the technology. The more complex a system, the more severely it is affected by its respective environment (and/or environments where there is interaction). As such, there may be a need to work backwards from what we are accustomed to, where we consider human impact assessments in a socio-technical context and add qualitative understanding to our quantitative approaches. This calls for a focus on qualitative approaches in addition to quantitative computational methods.Ā Ā
A useful and necessary first step would be for the international NMI community, in consultation with stakeholders across the ecosystem, to agree on what these characteristics are in the context of measurement, to promote the development and confident deployment of Trustworthy AI. Initiatives like the AI Standards Hub act as vital conveners where these frameworks and the metrics that may enable confidence in AI uptake can be agreed.Ā
Many frameworks already for measuring trustworthiness. As a first step those who wish to increase trustworthiness need to accept that one needs to make it easier for suppliers to comply with the frameworks that already exist, and also accept the code that drives systems AI is not static and therefore measurement tools cannot be static. Using this focus for discussion would be worthwhile.
Thanks for the comment Joseph, and agree with you on the need to ensure that not all tools are static. We need the right tools for different contexts and profiles.