Blog post by
Gill Jackson
Senior Researcher & Insight Manager, BSI
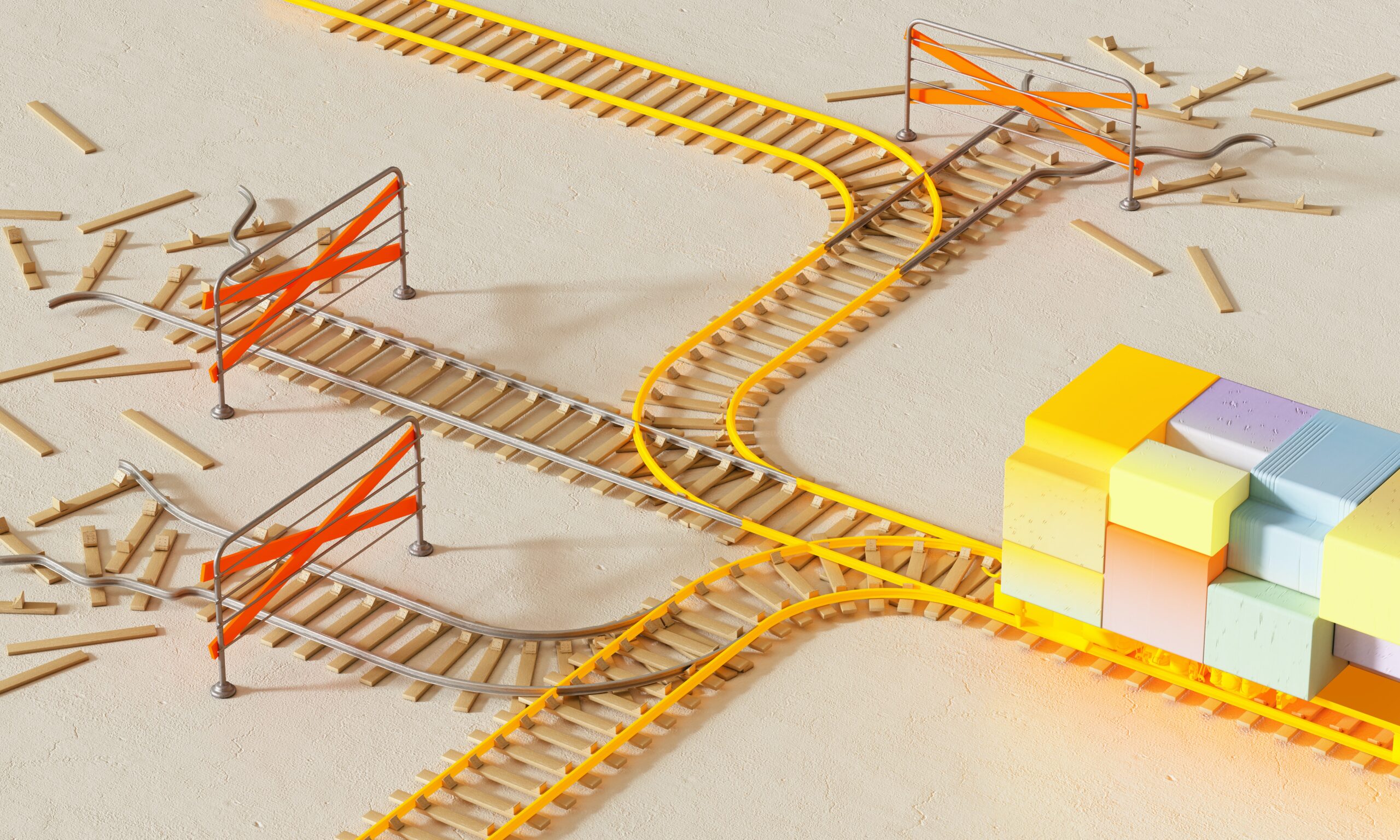
Since its launch last October, the AI Standards Hub has been exploring the theme of trustworthy AI with The Alan Turing Institute, BSI, and NPL each exploring a different aspect of this theme. BSI has led in exploring how standardization within the area of safety, security, and resilience for AI factors into the larger theme of trustworthiness. We have carried out interviews and held workshops with key industry stakeholders to better understand their approaches to ensuring AI is implemented in a safe, secure, and resilient manner, the barriers they encounter in doing so, and potential solutions to these barriers.
Safety and the basis of correlation
During our interviews we explored the definitions of safety, security, and resilience with participants. Here, āsafetyā was felt to be the most challenging to define. Participants felt the term could refer to the safety of the deployment of an AI system as well as the safety of the outcomes resulting from how the AI was used, each of which carrying its own risk factors.
An example from a US-based insurance provider illustrates this outcome-based safety concern well: because the dataset used to train the model captured a correlation between residentsā zip codes with certain levels of risk, people in certain areas were given poorer credit scores and denied loans. When considering AI safety, one must consider the basis of correlations on which a given AI system works. In this example, AI was used for the purpose of āactuarial fairnessā, but the question remains of whether the data point correlations justified using AI in the first place.
Security and resilience
Security was felt by those we interviewed to be a better understood area. This is particularly due to there being existing standards for cybersecurity. Participants felt that AI should not be treated any differently to other digital topic in relation to these standards. At the same time, AI raises unique security concerns related to the āfoolingā of AI systems, such as by āgamingā a self-driving carās safety mechanisms by placing stickers on stop signs or avoiding facial recognition by a person wearing masks or makeup.
The understanding of resilience in the context of AI proved more complicated. Robustness and repeatability were identified as key elements of AI resilience, and participants emphasised the requirement for consistency in a systemās outputs. However, when considering resilience, one must also consider other facets, such as cyber resilience, organisational resilience, human resilience, and information system resilience, as these would align with the usage and outputs of an AI system.
Challenges and solutions of AI implementation
A key challenge with AI is the question of applicability, both philosophical and technical. One can use AI systems in many contexts, however whether one should use an AI system in a given context is an important decision to consider up front. It is also important to consider Ā technical applicability, such as whether there is enough data to build an accurate model using machine learning techniques. These decisions can be guided by governance and policy frameworks, and here transparency is critical to tackle unrealistic fears and unrealistic expectations.
Another challenge is ensuring proper context: AI systems must be fit for their intended purpose and use case. Voice recognition systems, for example, may need to be trained on a wide range of potential languages, accents, and dialects they may encounter in deployment. Part of the solution to this challenge is to ensure that those procuring AI systems know what to ask for, and that developers likewise have the education and skills to ensure they can meet this need.
Our research also identified numerous low-level challenges, such as measuring the performance expectation of AI systems, ensuring the quality of testing and training data, addressing model bias, and ensuring adequate logging and monitoring. Here, standards can help guide developers and procurers on best practice in relation to each of these challenges.
Steps toward trustworthy AI
Our research and conversations identified a range of roles with differing needs when it comes to the deployment of AI systems. To ensure success, transparency around the specifics of the system is essential, as is the implementation of processes for human intervention. Here as well, standards can play an important role in defining acceptable performance.
As these considerations highlight, it is critical that stakeholders are knowledgeable about the standards landscape relating to AI. For developers, it is particularly essential to ensure they are able to meet existing standards as part of providing those looking to buy or use AI with assurance of systems meeting their requirements. We know from earlier research that stakeholdersā awareness of standards could be much stronger, and we are looking into potential solutions to improve this awareness, such as solution packs based on particular use cases. The Standards Database on the Hubās website is a good place to start exploring relevant standards, and we aim to continue improving and expanding this database as the ecosystem continues to develop.
0 Comments