Blog post by
Professor Mark Levene
Principal Research Scientist, NPL
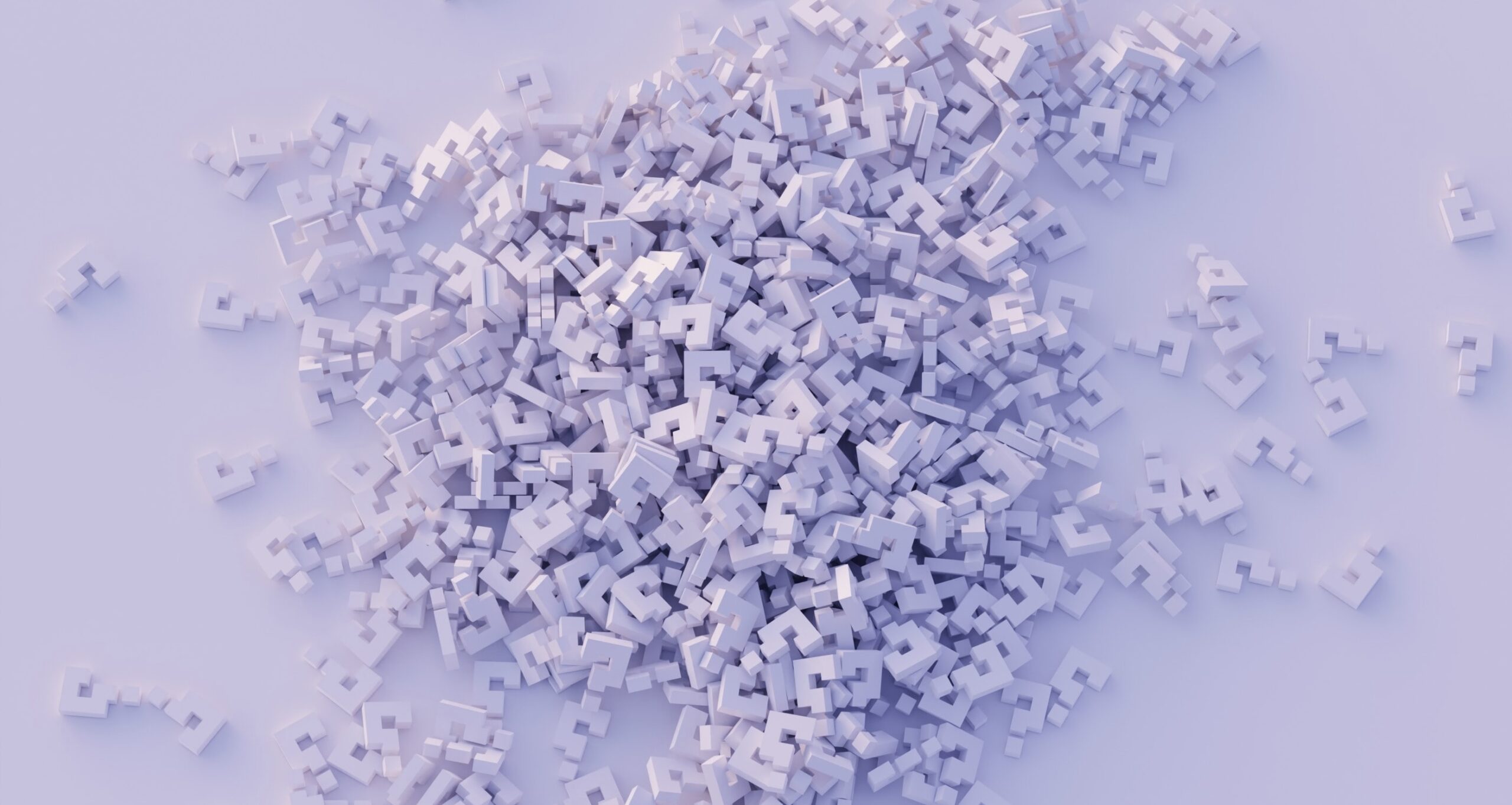
You are told by your weather app that it is going to rain tomorrow morning. Yet you have some doubt about this prediction, as it is not actually raining now. You look closer at the app and see a percentage, which you understand broadly as the chance that it will rain tomorrow. That is, the prediction of rain is attached to a statement of confidence: the higher the percentage shown in the app, the lesser the uncertainty (doubt) that it will actually rain.
From a technical perspective, we say that a measurementāin this case, resulting in the prediction that it will raināis incomplete without its associated uncertainty. Capturing this uncertainty is a central part of metrology, the science of measurement. (The term metrology is not to be confused with meteorology, the other scientific field behind your weather forecast.)
In the UK, the National Physical Laboratory (NPL) is the UKās National Metrology Institute and is responsible for developing and maintaining the national primary measurement standards. NPL also collaborates with metrology institutes around the world to maintain the international system of measurement.
Now that we understand what metrology is, what is this to do with artificial intelligence (AI) and machine learning (ML)?
As a small aside, let me clarify some terminology. Though the terms are quite often used interchangeably, AI is a more general term than ML, covering the theory and development of computer systems that are able to perform tasks normally requiring human intelligence. While AI includes symbolic computation such as pure rule-based systems, ML typically refers to AI computer systems that rely on a process of ālearningā from data and can have the ability to adapt. More specifically, ML builds statistical models of data that may be used for prediction and decision making.
Now, to answer the above question, the connection between AI and metrology lies in the fact that, in many cases, an AI system may be an important component of a complex measurement device. When we deploy an AI system, say to predict the weather, its output can be viewed as the result of a complex measurement conditioned on its inputāwhich, in this case, might include various physical measurements of the current state of the atmosphere.
Now that we have measurement in AI systems, there is also uncertainty which needs to be quantified, and we are thus in metrology territory. It naturally follows that one of the central problems that the Data Science Department at NPL is looking into is uncertainty quantification (UQ) in ML and AI. As discussed in a separate post, AI standards play a major role in communicating best practice in developing AI systems and more generally providing AI trustworthiness, topics which we will return to in future posts.
0 Comments