Blog post by:
Professor Mark Levene, Principal Scientist at the National Physical Laboratory (NPL) and Jenny Wooldridge, Senior Business Intelligence Analyst at NPLĀ
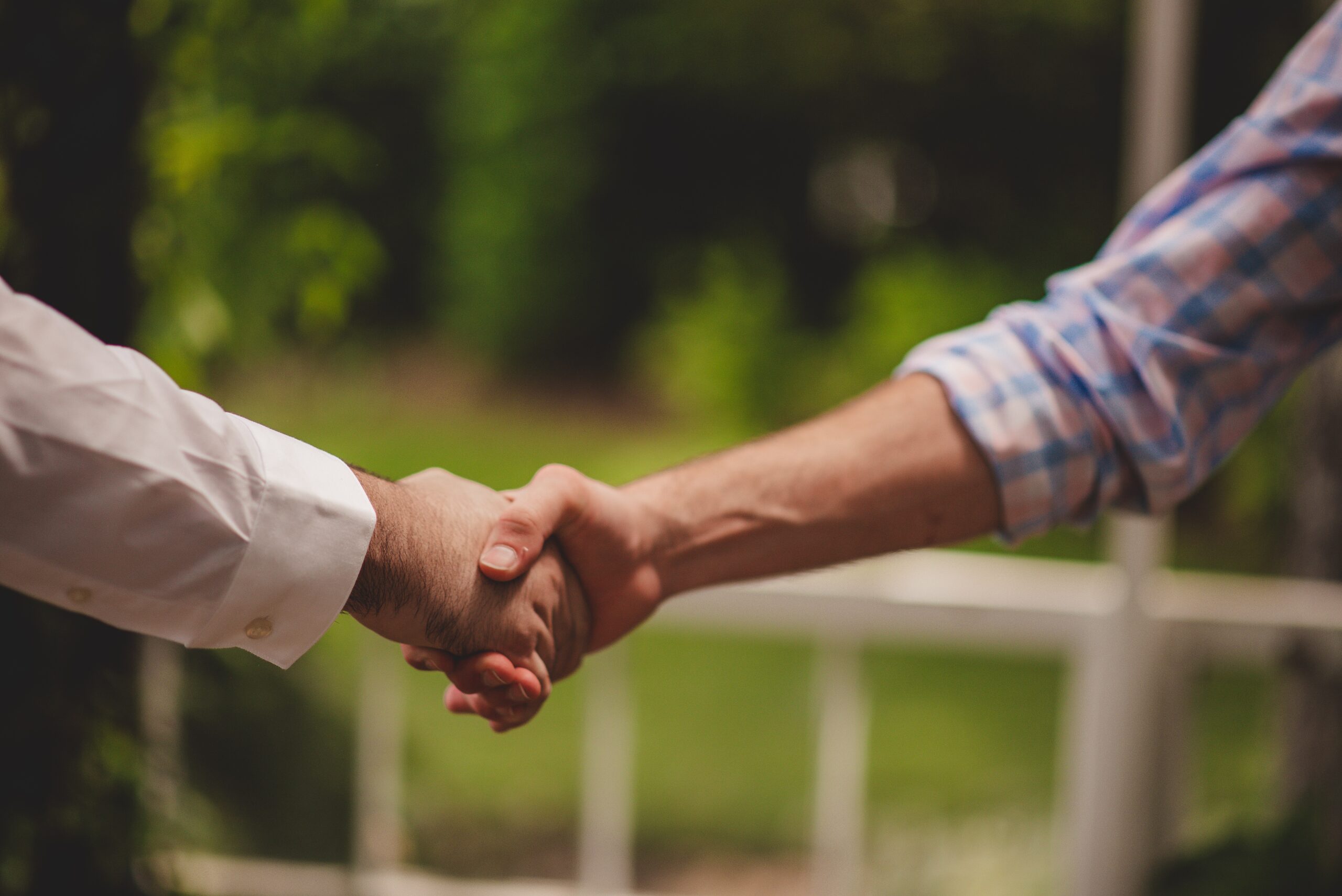
In a previous blog we introduced uncertainty quantification (UQ) in the context of AI and ML as a research area we are investigating at NPL. Here we discuss the important, but often overlooked, role that UQ plays in trustworthy AI.
The word ātrustworthyā can be understood as āable to be relied upon as honest, responsible and truthfulā. Trustworthy AI (TAI), or more specifically trustworthy ML, refers to the goal of ensuring that AI systems are “trustworthy” and can be relied upon to make responsible decisions. Thus, TAI can be viewed as a framework for managing risk and avoiding irresponsible use of AI systems. Apart from dealing with technical characteristics such as reliability, robustness, and resilience, trustworthy AI also considers sociotechnical characteristics such as explainability and freedom from bias and privacy, where human factors need to be taken into consideration. Moreover, issues involving ethics such as accountability, transparency and fairness are also essential to TAI.
UQ is a central concept in metrology, the science of measurement, and also for the characterisation of an AI system. This is because there is uncertainty both in the input data to the system (known as aleatoric uncertainty) and in the statistical model of the system that is used to make predictions (known as epistemic uncertainty). More technically, from a metrology perspective, uncertainty characterises the dispersion of values attributed to a measured quantity, and thus quantifies the degree of belief we have about the true value of that quantity. It should also be possible to trace data back to its source, as the uncertainty will propagate through the system from the input to the output and each link in the traceability chain will contribute to the uncertainty of the output of the system.
We are now ready to discuss the relationship between UQ and TAI. In a nutshell, by quantifying uncertainty about trustworthiness characteristics, we can be transparent about the limitations of an AI system under consideration. There are uncertainties associated with each step of the systemās engineering, from data preparation and model construction to model evaluation. From a userās perspective, communicating these uncertainties can provide a crucial layer that enhances the transparency of, and thus their trust in, the AI system.
As an example of the benefits of UQ, consider a medical practitioner making a diagnosis with the aid of an ML system that outputs a prediction in the form of a point estimate, which in this case may be generated from the result of a lab test. The guidance may be to deliver a positive diagnosis only if the estimate is above a threshold.
However, we recall that in metrology a measurement reported without an associated uncertainty is incomplete. Consequently, quantifying the output uncertainty (known as the predictive uncertainty) with an interval yielding a range of acceptable values below and above the estimate is much preferable to a point estimate.
Now, as long as the threshold is outside the prediction interval of the estimate, the practitioner can deliver a definite diagnosis. When the threshold is below the lower bound of the interval the diagnosis is positive, and when it is above the upper bound of the interval it is negative. However, when the threshold is included in the prediction interval, we cannot be certain whether it is below or above the estimate preventing the practitioner from making a definite diagnosis.
To summarise, predictive uncertainty equips the practitioner with the third option of āI am uncertain about the diagnosisā, pointing out that a second opinion is needed.
0 Comments